Machine Learning in Healthcare
Machine learning, a subset of artificial intelligence, involves the development of algorithms that enable computers to learn from and make decisions based on data. In the healthcare industry, machine learning is increasingly utilized to analyze electronic health records, patient data, and other clinical data to improve patient outcomes and streamline operations. Here are the applications of Machine Learning in Healthcare:
Electronic health records (EHRs)
Electronic health records are a critical source of patient information that can be leveraged to improve healthcare delivery. Machine learning enhances the usability and functionality of EHRs by providing deeper insights and predictive capabilities.
- Enhanced data management: Machine learning algorithms can automate the extraction and analysis of information from EHRs, identifying trends and providing insights to healthcare professionals for better clinical decisions.
- Predictive analytics: By analyzing health data, these algorithms can predict patient outcomes, such as the likelihood of hospital readmissions, allowing for preventive measures.
Patient data analysis
Patient data encompasses a wide range of information, including medical history, genetic information, and current health status. Machine learning effectively analyzes this data to enable more precise and personalized treatment approaches.
- Personalized medicine: Machine learning models analyze patient records and genetic data to create personalized treatment plans, improving the effectiveness of therapies.
- Disease risk assessment: Algorithms can identify patients at risk of developing certain conditions, enabling early intervention and improved management of chronic diseases.
Natural language processing (NLP)
Natural language processing allows computers to understand and interpret human language, making it highly valuable for processing clinical documentation and patient interactions. NLP can significantly reduce the administrative burden on healthcare professionals.
- Clinical documentation: NLP techniques can process and interpret unstructured data from medical records, streamlining the documentation process for medical professionals.
- Patient interaction: NLP-powered chatbots and virtual assistants provide 24/7 support to patients, answering queries and offering health advice based on their medical data.
Deep learning
Deep learning, a more advanced subset of machine learning, is particularly effective in processing complex data like medical images. Its applications in diagnostics and treatment planning are making significant strides in healthcare.
- Medical imaging: Deep learning models excel in analyzing medical images, such as X-rays, MRIs, and CT scans, aiding in the accurate detection and diagnosis of conditions like cancer and fractures.
- Diagnostic support: These models can provide preliminary diagnoses based on imaging data, helping radiologists and other specialists make more accurate decisions.
Medical research
Machine learning is crucial in advancing medical research. It processes large datasets to uncover new insights and accelerate discovery. It supports various stages of research, from hypothesis generation to clinical trials.
- Drug discovery: Machine learning accelerates drug discovery by predicting new compounds' efficacy and potential side effects, reducing the time and cost of bringing new drugs to market.
- Genomic research: Algorithms analyze genomic data to identify genetic variations linked to diseases, aiding in the development of targeted therapies.
Operational efficiency
Beyond clinical applications, machine learning also optimizes the operational aspects of healthcare, leading to better resource management and reduced operational costs. This contributes to a more efficient and responsive healthcare system.
- Resource allocation: Machine learning optimizes hospital resource allocation, including staff scheduling and inventory management, enhancing operational efficiency and patient care.
- Predictive maintenance: By predicting when medical equipment will likely fail, machine learning ensures timely maintenance, minimizing downtime and ensuring critical devices are always available.
Remote monitoring and telemedicine
With the rise of telemedicine and remote patient monitoring, machine learning offers significant benefits in continuous health monitoring and virtual care delivery. It supports healthcare professionals in providing timely and effective care.
- Wearable devices: Machine learning processes data from wearable devices to monitor patients' vital signs in real-time, alerting healthcare providers to potential issues before they become critical.
- Telehealth support: ML enhances telemedicine platforms by providing decision support tools that assist healthcare professionals in diagnosing and treating patients remotely.
By integrating machine learning into various aspects of the healthcare industry, medical professionals can leverage these advanced technologies to enhance patient care, improve diagnostic accuracy, and streamline operations. As the adoption of machine learning continues to grow, its potential to transform healthcare becomes increasingly evident, making it an essential tool for modern medical practice.
The impact of Machine Learning in Healthcare
Machine learning profoundly impacts the healthcare industry, offering numerous benefits that enhance patient care, streamline operations, and advance medical research. Integrating machine learning technologies revolutionizes how healthcare organizations manage and utilize data, leading to significant improvements across various domains. These are the benefits of using Machine Learning in Healthcare:
Precision medicine
Precision medicine aims to tailor treatments to individual patients based on their unique characteristics, such as genetics, lifestyle, and environment. Machine learning facilitates this approach by analyzing vast healthcare data to identify patterns and predict treatment responses. This personalized approach enhances the effectiveness of therapies and minimizes adverse effects, leading to better patient outcomes.
Advanced diagnostic capabilities
Deep learning models and neural networks excel at processing complex data, such as medical images. These models can accurately detect abnormalities in X-rays, MRIs, and CT scans, supporting radiologists in diagnosing conditions like cancer, fractures, and neurological disorders. This enhances the accuracy and speed of diagnoses, ultimately improving patient care.
Machine learning algorithms also assist in planning and optimizing medical procedures, ensuring they are performed precisely. This reduces the risk of complications and improves the overall quality of surgical and other medical interventions.
Enhanced data collection and management
Efficient data collection and management are crucial for healthcare systems to function effectively. Machine learning streamlines the process of gathering and organizing healthcare data from various sources, including electronic health records, wearable devices, and medical research. This ensures that healthcare professionals access comprehensive and up-to-date information, enabling better decision-making and improved patient care.
Pharmaceutical innovations
Pharmaceutical companies leverage machine learning to accelerate drug discovery and development. By analyzing biological data and predicting the efficacy and safety of new compounds, machine learning models significantly reduce the time and cost associated with bringing new drugs to market. This leads to faster availability of innovative treatments for patients.
Optimized healthcare operations
Machine learning optimizes healthcare organizations' operations by improving resource allocation, staff scheduling, and inventory management. This results in more efficient resource use, reduced operational costs, and enhanced patient care.
In addition, machine learning enhances the coordination and delivery of services, ensuring that patients receive timely and appropriate care. This improves overall system efficiency and patient satisfaction.
Improved medical records management
Managing medical records efficiently is essential for providing high-quality patient care. Machine learning algorithms automate the extraction, organization, and analysis of information from medical records, making accessing relevant data easier for healthcare professionals. This reduces administrative burdens and allows medical staff to focus more on patient care.
Challenges and Ethics of Machine Learning in Healthcare
Machine Learning in Healthcare has the potential to revolutionize patient care by improving diagnosis, treatment, and patient outcomes. However, integrating machine learning technology into healthcare services also raises several challenges and ethical concerns that must be addressed to ensure these systems' safe and effective use.
Data quality and collection
Data quality is critical for machine learning models to perform accurately. Poor data quality can lead to inaccurate predictions and poor patient outcomes. Data scientists must ensure that the data used to train machine learning models is accurate, complete, and representative of the population being studied.
Historical data can be biased and may not reflect the current medical landscape accurately, leading to inaccurate predictions and poor patient outcomes.
Clinical decision support
Machine learning models must be integrated into clinical practice to ensure their effective and safe use. Clinical expertise is necessary to ensure that machine learning models are used correctly and do not replace human judgment and decision-making. Machine learning models can improve clinical workflows by automating routine tasks and improving the accuracy of diagnoses.
Early detection and improved patient outcomes
Machine learning models can detect diseases early, improving patient outcomes and reducing healthcare costs. Improved patient outcomes can be achieved by providing personalized treatment plans and improving the accuracy of diagnoses. Machine learning models can be used in various healthcare applications, including diagnosis, treatment, and patient care.
Robotics and surgical applications
Robotics and robotic surgery can improve patient outcomes by providing more precise and minimally invasive procedures. Machine learning models can improve surgical outcomes by providing real-time feedback and guidance during procedures. Healthcare institutions must ensure that machine learning models are developed and implemented correctly to ensure their safety and effectiveness.
Healthcare services and clinical workflows
Machine learning models can improve healthcare services by streamlining clinical workflows and patient care. Healthcare applications of machine learning include diagnosis, treatment, and patient care. Machine learning engineers must ensure that machine learning models are developed and implemented correctly to ensure their safety and effectiveness.
Ethics and challenges
Machine Learning in Healthcare raises several ethical concerns, including data privacy, bias, and the potential for machine learning models to replace human judgment and decision-making. Challenges include data quality, clinical decision support, and the integration of machine learning models into clinical practice. Human intervention is necessary to ensure machine learning models are used correctly and not replace human judgment and decision-making.
Carepatron: Rewriting the future of healthcare
Carepatron is transforming the healthcare landscape with its innovative practice management software and virtual medical scribe solutions. By integrating advanced machine learning technologies, Carepatron streamlines administrative tasks, allowing healthcare professionals to focus more on patient care.
Its virtual medical scribe efficiently captures and organizes clinical data, reducing doctors' documentation burden and improving medical records accuracy. This holistic approach enhances healthcare operations' efficiency and contributes to better patient outcomes, positioning Carepatron as a key player in the future of healthcare management.
Discover how Carepatron's innovative solutions can streamline your practice and enhance patient care—try Carepatron today!
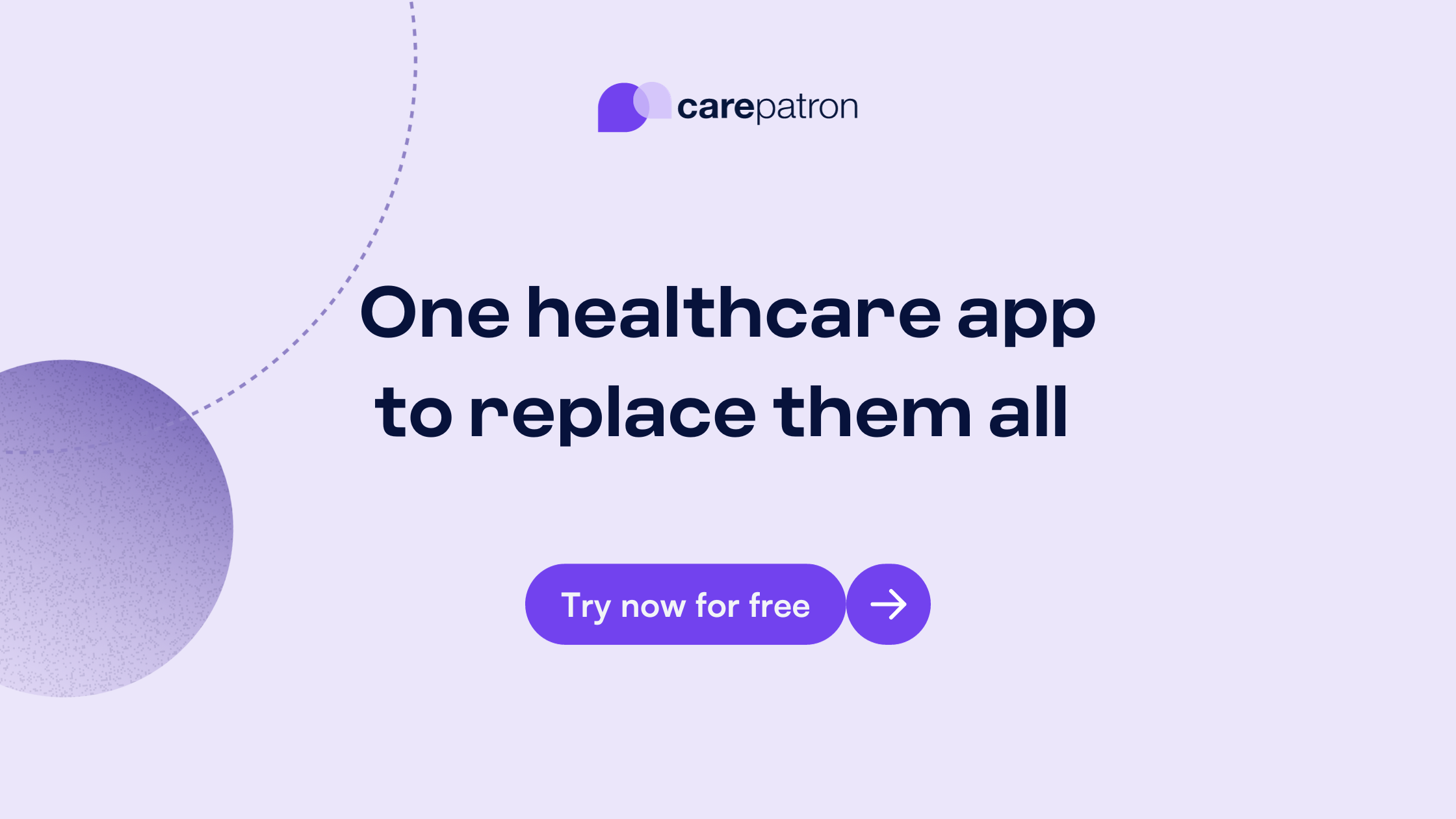